Dr. Peter Dayan Group Talk
- Datum: 09.05.2025
- Uhrzeit: 11:00 - 12:30
- Vortragende(r): Tianyuan Teng, Sahiti Chebolu, Azadeh Nazemorroaya
- Ort: MPI for Intelligent Systems, Max-Planck-Ring 4
- Raum: Room 203 + Zoom
- Gastgeber: Dr. Peter Dayan
- Kontakt: wenting.wang@tuebingen.mpg.de
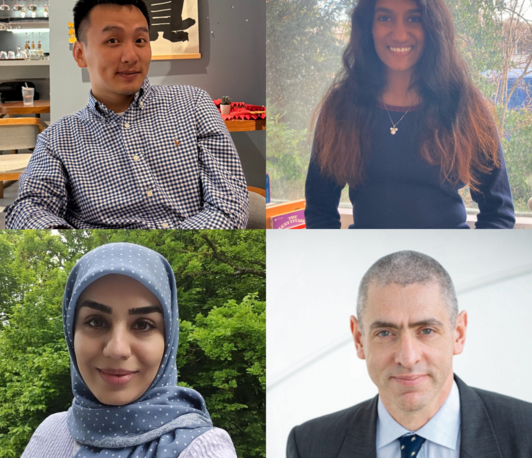
Tianyuan Teng - Meta-decision making about hierarchical planning
Abstract: Hierarchical planning (HP) enables efficient action selection by leveraging structured representations and computations, but this efficiency comes at a potential cost: abstraction can lead to information loss and suboptimal decisions. We investigated whether human meta-decision-making is sensitive to this trade-off when choosing between hierarchical and flat planning strategies. In two preregistered experiments, we tested whether participants were more likely to commit to hierarchical plans when facing (1) more remote goals, (2) greater environmental complexity, (3) limited planning resources, and (4) increased environmental uncertainty. All hypotheses were supported. To formalize this behavior, we developed a meta-decision-maker model that quantifies the utility of different representational granularities and accounts for diverse human navigation patterns. Our findings highlight the human capacity to flexibly adjust planning granularity, thereby reducing computational demands while maintaining adaptability to new information.
Sahiti Chebolu - Weighting waiting: Optimal and sub-optimal inter-temporal decisions explain delays in a real-world task
Abstract: People often distribute work over time in diverse patterns, sometimes procrastinating at a cost to well-being. While many factors behind delay are known, mechanistic explanations remain limited. We explore decision-making policies for delay using reinforcement learning models, developing a taxonomy of pacing and procrastination. We illustrate the taxonomy through simulations of some of the mechanisms and assess the operation of them in a real-world task (using a rich dataset published by Zhang & Ma, Scientific Reports, 2024), showing that each of these mechanisms constitute a plausible candidate for students’ decisions to delay.
Azadeh Nazemorroaya - State-independent Action learning
Abstract: Adaptive learning involves repeating actions that previously led to desirable outcomes. However, such learning can become maladaptive when individuals ignore state information critical to successful decision-making. This phenomenon, known as state-independent learning, has been linked to compulsivity and reduced working memory, suggesting that both trait-like and cognitive resource limitations may drive reliance on simplified, context-free strategies. Yet, state-independent learning is not inherently maladaptive. In some cases, it may reflect an efficient heuristic or an adaptive response to noisy environments. Understanding when and how this strategy emerges remains an open question, particularly in emotionally charged or motivationally salient tasks. We examined a large sample (N=817) from a Motivational Go/NoGo task to assess how frequently and strongly state-independent learning influences behavior. In this task, participants learn to perform or withhold actions in response to cues indicating reward or punishment. While action learning is typically state-dependent, Pavlovian biases and state-independent tendencies may interfere. Using both behavioral analysis and computational modeling, we investigated the extent to which participants relied on recent action-outcome histories across states, revealing the prevalence and potential adaptive role of state-independent learning in this context.
Lab's webpage: https://www.kyb.tuebingen.mpg.de/computational-neuroscience
Access to the meeting: Zoom Link