Cybernetics and neural systems
The intertwined research journey of AI and neuroscience
Cybernetics' big breakthrough was realizing that complex systems, whether machines, biological organisms, economies, or societies, are driven by feedback loops of information. The insight would prove particularly instrumental in two fields that were just starting out in the 1950s: neuroscience and computer science. Researchers in both these areas not only utilized the new understanding to propel innovations in their respective areas. Neuroscience and computers also became interwoven in ways that might deeply shape humanity’s future.
For one thing, the neural circuits of the brain became a guiding model for AI development. For another, neuroscientists are now exploring ways to incorporate machine thinking into the brain, leveraging AI technologies.
Early cyberneticists, such as John von Neumann, had predicted that a computer could simulate the cognitive processes of the human brain. One of the first to prove him right was the American psychologist Frank Rosenblatt, who developed a simple "neural network" machine called the Perceptron in the 1950s. It was based on an earlier realization by neuroscientists that the brain stores memories and learning by strengthening or weakening synaptic connections between individual neurons.
The Perceptron: one of the first artificial neural networks
Rosenblatt's Perceptron used the same approach, adjusting connection strengths between nodes in an electrical circuit to learn and classify simple patterns. This strategy is still employed in today's large language models, where connections between digital nodes are adjusted through learning algorithms.
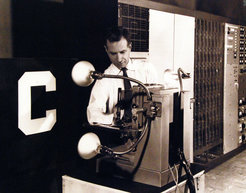
Other cyberneticists, like Britain's Grey Walter, built small robots that operated via brain-like circuits. Walter's famous artificial tortoises – each constructed from two valves, two relays, two motors, two condensers, and a sensor for either light or touch – learned simple tricks such as approaching at the sound of a whistle.
Computer scientists also looked to the brain to teach machines to "see." How, for example, can a computer distinguish if a picture shows a cat, an apple, or a bridge? Neuroscientists in the 1950s and 1960s found that the brain builds up complex images by detecting basic building blocks such as edges, using special nerve cells. This insight served as the foundation for developing computer networks, which excel at recognizing patterns in images by breaking them down into simpler elements, mimicking the brain's visual processing.
Machines learn differently than humans
Similarly, computer scientists drew upon brain mechanisms to create algorithms for focusing attention in artificial systems, reinforcing learning, and building complex hierarchical representations of data.
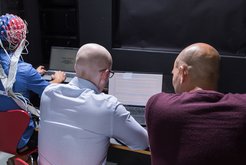
It would take decades before processors became powerful enough for complex applications such as efficient speech recognition or text processing. (Walter's rudimentary tortoises operated with the equivalent of just two neurons.) Even today's impressive large language models do not reach the full capabilities of the human brain. They can beat humans at certain tasks such as pattern recognition or data analysis because they process information via massive parallel networks (as opposed to the step-by-step, sequential process of neurons in the brain). But their learning is fundamentally different.
Humans can rapidly learn new information from just a single exposure and solve novel problems they haven't encountered before. In contrast, AI systems require extensive training on hundreds of repetitions of the same data to learn and often struggle with situations unfamiliar to their training data. An AI trained to recognize cars with the help of photos taken on sunny days might not recognize a car covered in snow, for example.
The brain also works much more efficiently, consuming only about 20 watts (less than half a laptop´s power consumption) while AI systems consume hundreds of thousands of times more energy to run.
Nevertheless, these artificial networks are now producing neural activity patterns that so closely resemble what happens in biological brains that neuroscientists have started to use these computer systems as stand-ins to test out theories about how our real brains work. AI has also become a powerful tool to analyze the vast datasets that neuroscientists gather and to extract patterns from them.
Integration of artificial and biological intelligence
In the newly emerging field of neuro-cybernetics, neuroscientists are even developing brain-computer interfaces (BCIs) that directly integrate biological brains with machines. In the first applications, neurosurgeons have, for example, implanted wires into the brain of paralyzed patients that translate neural activity into movements of robotic arms or legs. Other non-invasive BCIs measure a user's brain signals from outside the skull and use it to determine whether to mute, for example, email notifications while the user is focused on work. Though still largely experimental, BCIs might also soon start to enable mind-controlled operation of smartphone apps, smart home devices, and even drones for hands-free use.
Basics of Brain-Computer Interfaces
Since May 2023, around 50 experts from biomedicine, neuromechanics, neuroscience, computer science, and other fields have been working in Germany to develop bionic systems, which are systems that bridge the gap between biological structures and technical applications. Known as BITS, short for the Center for Bionic Intelligence Tübingen Stuttgart, this interdisciplinary research initiative is part of Cyber Valley, Europe's largest research cooperation for robotics and artificial intelligence. The BITS projects range from robotic orthoses to wearable sensors that can be used for obsessive-compulsive disorders to new forms of brain stimulation.
Looking ahead, neuro-cybernetics may lead to brain implants that could enhance human cognitive abilities such as memory and learning. This would bring full circle the original cybernetic postulate from the early 20th century – that biological and artificial systems fundamentally operate in the same way.